Understanding Key Factors Influencing Airbnb Success in Madrid
Starting as an Airbnb host in Madrid can be challenging. How can you make your property stand out? Is location the key to maximizing your return on investment? This project explores key factors that influence your property's perceived value among potential guests. Discover how these insights can elevate your hosting game to the next level
5/8/20247 min read
Airbnb, the global online marketplace for short- and long-term stays, offers a wealth of accommodation options across various regions, including Madrid. As Spain’s central capital, Madrid boasts 21 districts and 131 neighborhoods, with a bustling population of 3.2 million residents—expanding to 6.55 million in the surrounding community. Despite the city's size and diversity, nearly 50% of Airbnb's 142,000 listings are concentrated in the Centro district, which includes popular neighborhoods like Embajadores, Universidad, Palacio, Cortes, Sol, and Justicia
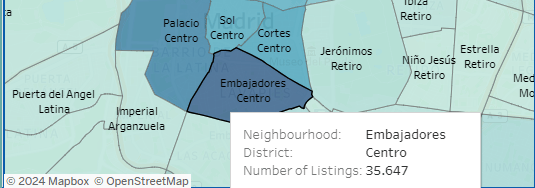
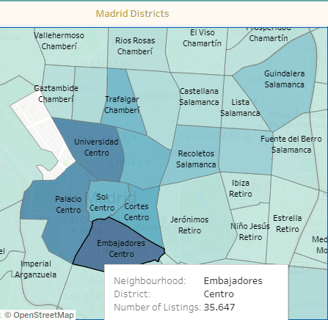
Objectives
Image showing the listings distribution in Madrid Centro district. From Tableau dinamic dashboard
As a new Airbnb host, it might seem that the Centro district is the only viable area for business. But do we truly understand the various factors affecting a listing’s visibility on Airbnb? How much weight does location carry compared to other elements? And what other factors might influence Airbnb's algorithm?
This analysis aims to explore the distribution of listings across Madrid to pinpoint areas where competition may be fierce or where growth opportunities exist. Additionally, we will delve into factors beyond location that shape clients' perceptions of value, as these can be crucial revenue drivers for new hosts.
Project background
Tools
Datasets
Limitations
Anaconda/Jupiter Notebook
Pandas
Data wrangling & Subsetting
Consistency checks
Combining, Deriving • Data clustering
Visualizations: created using matplotlib
Statsmodels.api: statistical modeling
Sklearn: machine learning, data analysis
The data is collected on a quarterly basis for the past 12 months. Historical data beyond this period is not included, which limits the ability to analyse long-term trends over time.
The data behind the Inside Airbnb site is sourced from publicly available information from the Airbnb site.
Data Analysis
In this data analysis, we take a systematic approach to uncover insights that can help new Airbnb hosts in Madrid maximize their property's performance.
Step 1
Cleaning and merging multiple datasets to ensure accuracy, using pandas for data wrangling, aggregation, sklearn for linear regression modelling and clustering using Kmeans and statsmodels.api for a time series analysis ( stationary tests)
Conduct an exploratory analysis to understand the distribution of market listings and identify factors that correlate with or impact pricing. Use clustering to group listings and detail the behavioral characteristics of each cluster.
Step 2
The results are in alignment with Airbnb's main SEO visibility factors, providing actionable recommendations to enhance listing rankings and drive growth
Step 3
The heatmap annotation allows us to identify the correlations coefficients of the variables we will use as part of the study. Here are some of them:
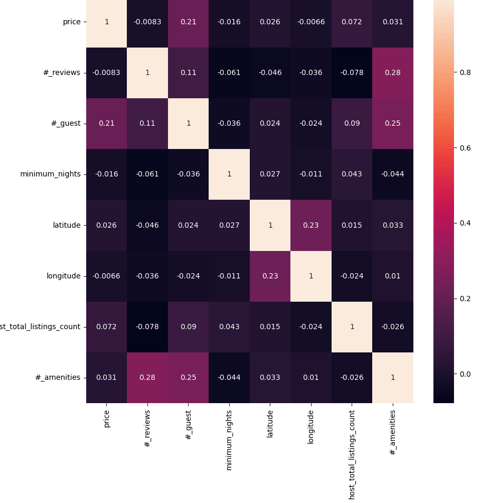
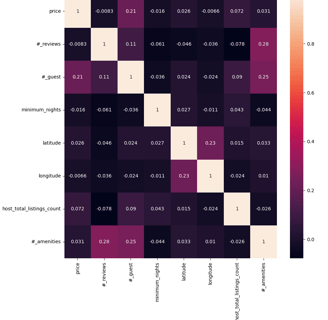
#_amenities and #_guest
Suggesting that the higher the number of guest the more needs they might have and therefore more amenities the accomodation must have.
#_amenities and #_reviews
Indicating that when the accomodation has a higher number of amenities the listing tends to get more reviews.
price and #_guest:
Implying that as the capacity for guests increases, the price tends to be higher, which is logical since larger accommodations typically cost more.
Upon Airbnb´s listings distribution in Madrid location seems to be a key factor for users. However, data reveals that listings with a higher location score have a moderate correlation coefficient versus pricing.
(Note: When focusing on the average price range (80€ to 180€), location score appears to play a meaningful role in determining price, resulting in an upward trend. However, when expanding the price range to include extremely high-priced listings (up to 21,000€ ), the influence of location score diminishes, leading to a flatter trend line. This suggests that at extreme prices, other factors beyond location become the primary drivers of pricing, thus diluting the impact of location score on the overall correlation.)
Data Insights
Setting these anomalies aside, the exploratory analysis revealed that the scatter plot’s trendline showed only a moderate relationship, indicating that other factors have a greater influence on pricing. This insight prompted a shift in the focus of the analysis.
Although the dataset contained many variables, the heatmap helped narrow down the most impactful ones:
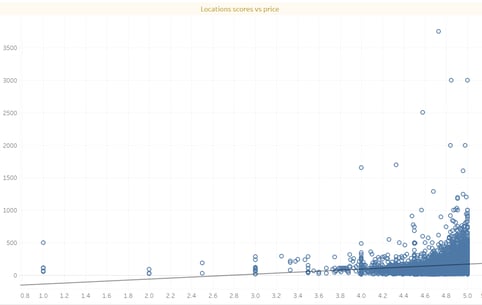
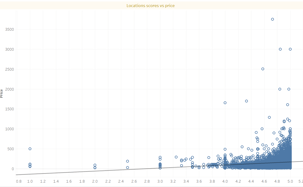
Despite the concentration of listings in the Centro district, a choropleth map revealed that higher-priced listings were also present in less typical areas like Carabanchel, Arganzuela, Tetuán or Chamartín where the number of listings is quite limited. Initially, I assumed these might be long-term rentals, but upon closer inspection, I was surprised to find that the prices were actually listed on a per-day basis. This means that the price range of these some of this appartments might vary between €35K to €100K a week.
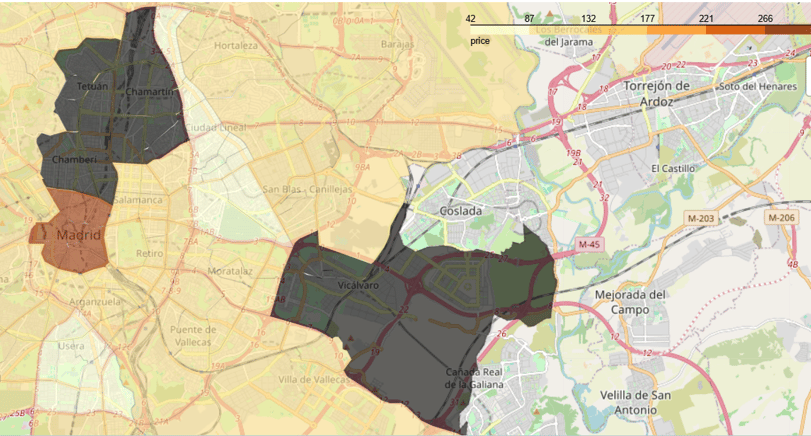
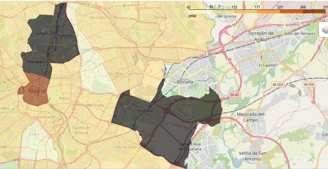
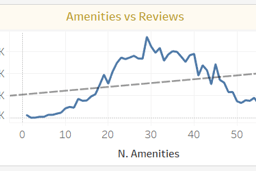
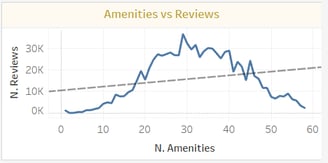
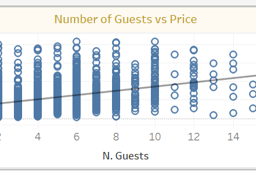
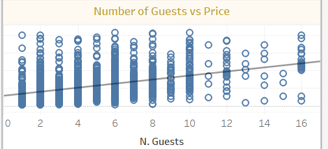
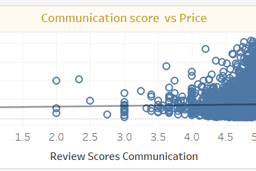
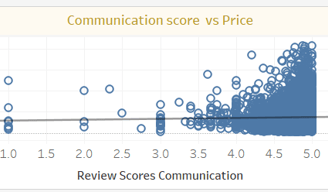
These images are a selection of example graphs from regression analysis completed. (access full report)
The regression analysis provided a clearer understanding of the relationship between these variables and price. To segment the data for deeper insights, I used the elbow curve method to determine the optimal number of clusters. This approach allowed for a more detailed exploration of the behaviors and characteristics influencing pricing.
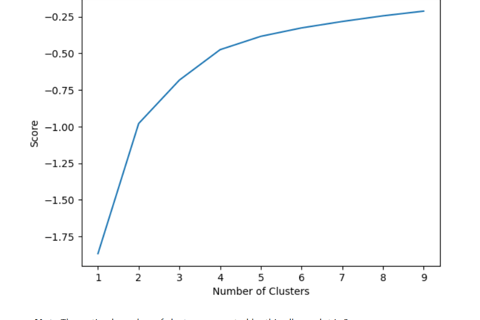
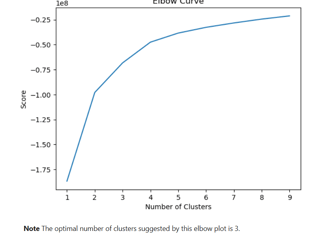
Note: The optimal number of clusters suggested by this elbow plot is 3
Number of amenities
Reviews scores
Cleanness scores
Communication scores
Number of guests
Cluster Analysis
Now that we’ve identified additional factors influencing listing prices, let's break them down into clusters to uncover common patterns and behaviors.
Below are the results of the clustered regression analysis, highlighting the distinct groups and their varying characteristics.
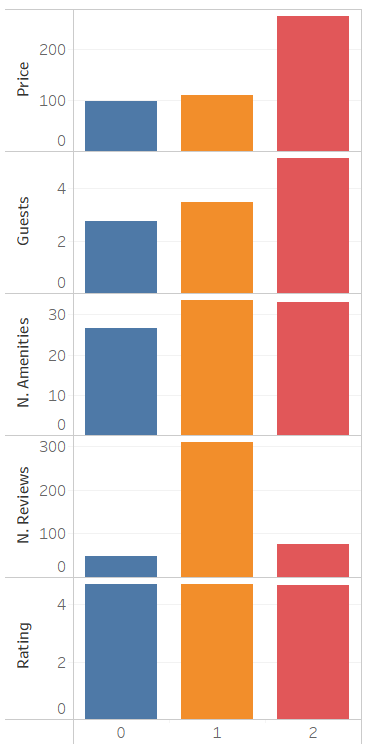
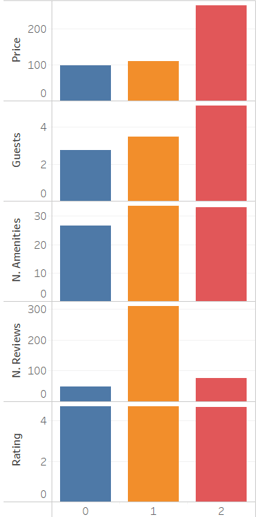
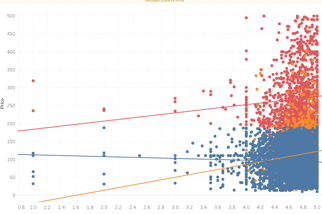
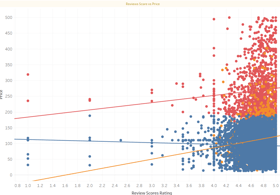
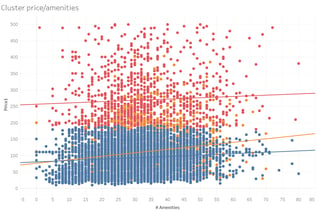
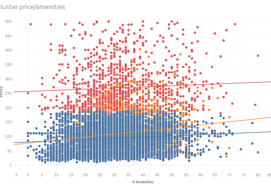
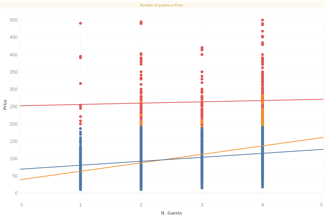
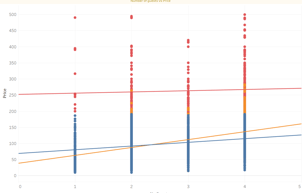
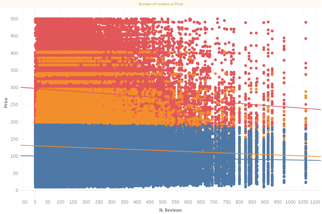
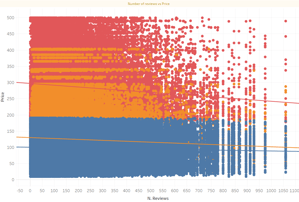
The Red cluster appears to be more luxurious or spacious properties, offering accommodation for more guests at a higher price. Despite having a similar number of amenities as the Orange cluster, the ability to host more guests may justify the price premium.
The Orange cluster seems to have high popularity (highest number of reviews) and customer satisfaction (highest review scores) but is priced in the mid-range. This could indicate that these listings are priced competitively or offer good value.
The Blue cluster offers the lowest number of amenities and guest capacity, correlating with its lower price. However, it maintains high review scores, suggesting a value-for-money proposition where customers are satisfied despite lower prices and amenities.
Recommendations
Positive reviews and ratings not only impact guest booking decisions but also play a crucial role in your Airbnb SEO.
Airbnb SEO involves optimizing your listing to increase its visibility in search results when potential guests browse for accommodations. While Airbnb hasn’t fully disclosed its search algorithm, it’s known to consider various factors, including price, location, amenities, reviews, response rates, booking acceptance, and cancellation history.
The platform employs a machine learning system that analyzes past bookings and searches to predict what a user is most likely to book. Therefore, a well-optimized listing with accurate details and positive guest experiences is more likely to rank higher in search results
Let's break down the three key factors that can boost your ranking:
Quality
Offer Competitive Amenities
Adding more amenities to your property can increase your visibility because guests often filter their searches by amenities. The average number of amenities is 26.
However, according to Airbnb, the top 10 amenities guests filter for are:
A pool
Wi-Fi
A kitchen
Free parking
A hot tub
A washing machine or dryer
Air conditioning or heating
Self check-in
Laptop-friendly workspace
Pets allowed
Popularity
Provide Exceptional Hospitality
Airbnb data shows guests are more likely to book a listing with a high rating. And those ratings also play a role in a listing’s ranking in Airbnb search results.
Use the specific categories that guests rate as guidelines for improvements.
These categories include:
Check-in: Make check-in quick, easy, and clear for guests
Cleanliness: Keep your property in the clean and tidy condition shown in the listing photos
Accuracy: Ensure features, facilities, and amenities are exactly as described in your listing
Communication: Communicate quickly and clearly with guests, responding promptly to any issues
Value: Price your listing fairly to avoid dissatisfying guests
Price
Offer Competitive Pricing
Airbnb prioritizes listings that are priced below comparable ones.
So, set a competitive price compared to similar listings nearby to increase your visibility and drive more bookings.
You can test pricing changes or discounts to see how they impact bookings.
Alternatively, you can let Airbnb do the work for you by turning on Smart Pricing. This feature automatically changes the price of a nightly stay depending on demand.
You can set upper and lower limits to Smart Pricing to have more control.
If you have a listing in Madrid, there are growth opportunities in many districts and neighborhoods.
Having a good visibility of your listings in Madrid Centro can be a challenging task due to the high number of listings currently competing.
However, other districts may offer a better competitive advantage, allowing you to highlight your listing based on pricing, amenities, or the quality of the accommodation.